Assessment Movement, ChatGPT3, and some Muscle Physiology
Happy (late) New Year :)
🦾 Biomechanics, motor control, and (re)training paper recommendations
Association Between Passive Hip Range of Motion and Pitching Kinematics in High School Baseball Pitchers

Practitioners commonly assess the passive range of motion of various joints as they assume limitations in joint ROM may impact how people move during their sport. While this is a logical hypothesis, this paper provides yet more data that the relationship between peoples' passive ROM and sports performance is not linear. In their paper, only the passive internal rotation ROM of the drive leg was moderately related to the trunk rotation angle during a pitch, with an R2 = 0.177 (i.e., passive hip ROM explains about 18% of the variance in trunk rotation angles during pitching). Thus, this data supports previous findings that caution should be advised if using only passive ROM measures to make inferences about how people may move during their sport.
Comparison of OnBaseU Tests with Biomechanical Motion Analysis in Youth Baseball Pitchers

Continuing on the movement assessment theme, this paper further outlines the difficulty associated with designing movement assessment protocols that can yield insights into how people move beyond the gym and in their sport.
The predictive value of general movement tasks in assessing occupational task performance
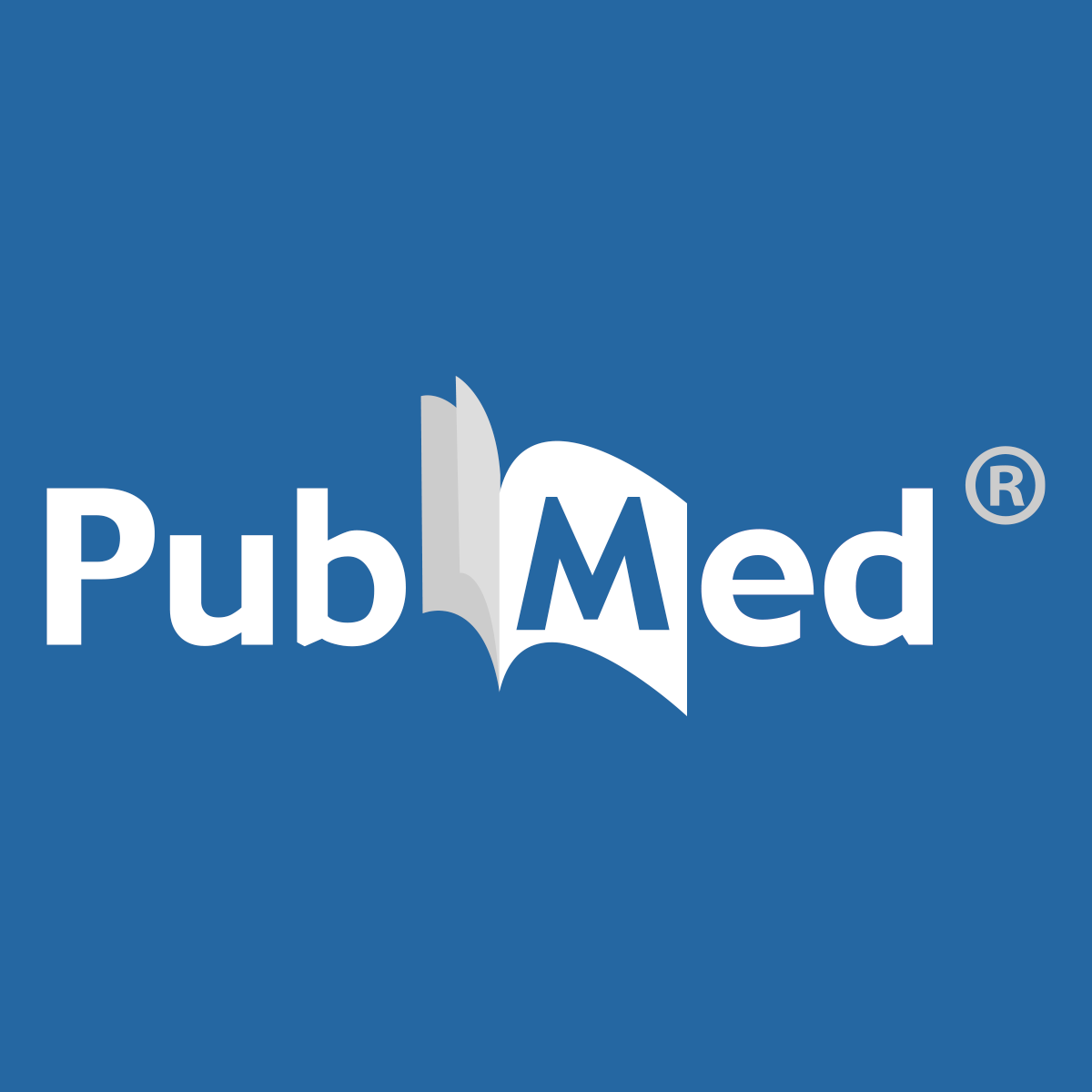
To provide a bit more of a positive spin to the perhaps "negativity" of the previous two papers, it seems as though increasing the demands of the squats during a movement assessment (e.g., increasing the loads people move with, the speeds in which they move, or both) can provide perhaps more insight regarding how firefighters may perform simulated fireground tasks. More research is certainly required in this space to understand how to assess movement behaviours that may translate to sport or work, but this hints that many of the tasks included in low-demand movement assessments that practitioners typically default to can perhaps become more informative simply by elevating the demands.
🤖 Statistics and machine learning paper recommendations
The development and evaluation of a fully automated markerless motion capture workflow
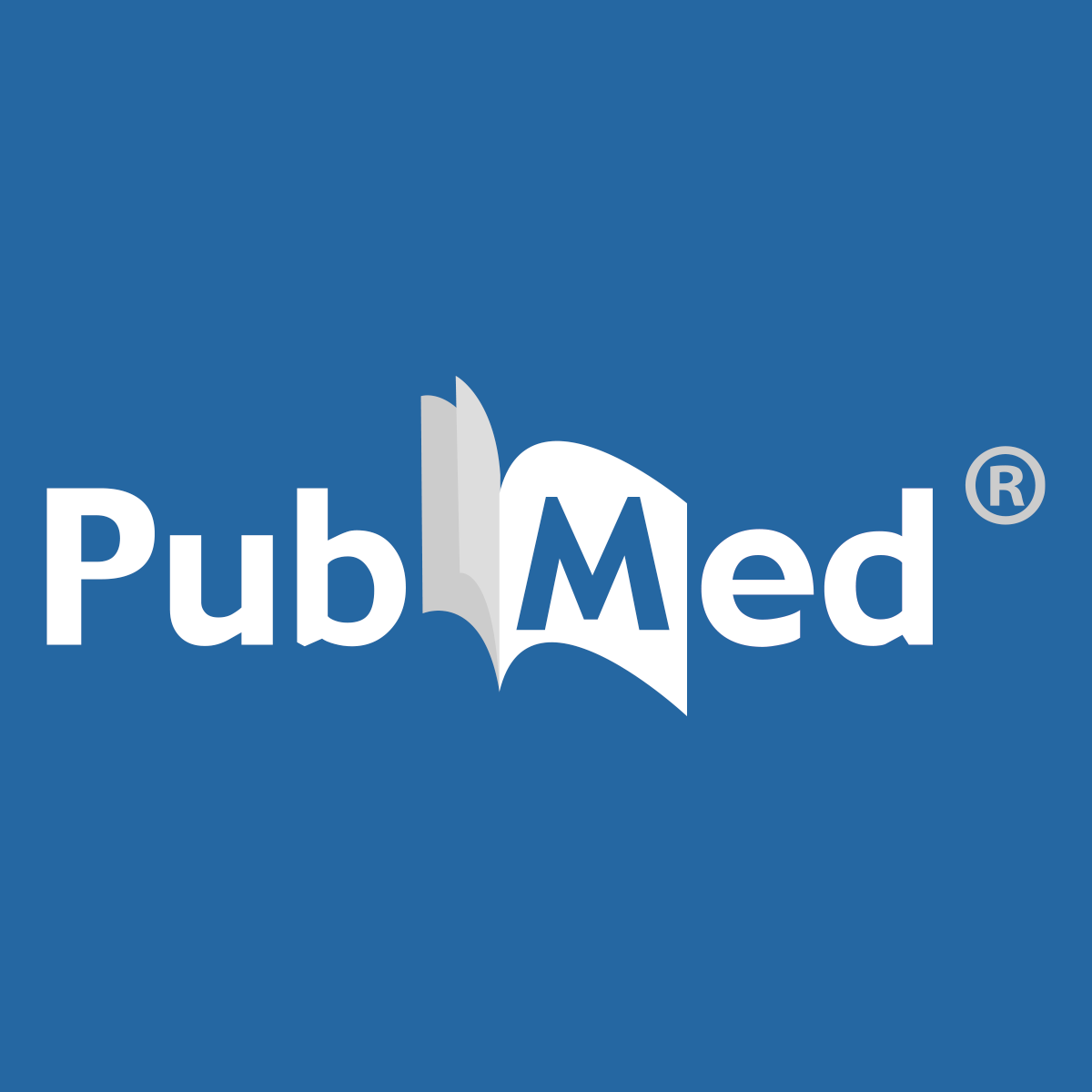
Another example of how markerless motion capture workflows are becoming more ubiquitous in biomechanics. When there is time, it would be great to add something like this to the Kinect v2 repo I put together a while ago (or, since it's open source, someone else could tackle that as a fun side project!).
Chat GPT3
For people who aren't familiar with or have heard about it, I highly recommend becoming familiar with emerging technologies such as Chat GPT3 (try it out for yourself!). It is a tool where you can seemingly ask anything and provide an accurate text-based answer. So accurate that it recently passed the Wharton MBA exam and the US test for a medical license. What's also really interesting about it is that it's just a "base" upon which you can build other models. For example, someone made an Andrew Huberman bot (trained on the audio from his podcasts, some of which I've shared on this newsletter) where you can ask it questions and it provides text-based answers as though it's Dr. Huberman himself.
Of course, rather than treating it as an ever-knowing entity, it shines as a tool used alongside humans:
A simple algorithm to decide whether to use ChatGPT, based on my recent article (https://t.co/tTzD0RAM0M) pic.twitter.com/rs9vr1lAvI
— Aleksandr Tiulkanov (@shadbush) January 19, 2023
As these technologies become more popular, using them to assist with topics in which you have the expertise to verify an accurate output (and just needed Chat GPT3's help to constrain the problem slightly) will surely help boost your productivity while improving the quality of your work.
Of course, there's tons of ML-related content to geek out on with models such as these, but I think the practical applications are worth highlighting.
If you're interested in other similar tools/models, check out the following LinkedIn post:
Commentary: Artificial Intelligence and Statistics: Just the Old Wine in New Wineskins?

This is an interesting commentary as it succinctly highlights the errors that may arise when evaluating prediction models based on more "classical" ML-based evaluation techniques (e.g., F1 score) rather than focusing on a model's discriminative ability or clinical usefulness. Highly suggest reading this if you are interested in creating predictive models.
🎙 Podcast recommendation
I enjoyed this chat between Peter and Andy, especially as it helped brush me up on my physiology (which really needs a refresher).
🗣 Quote of the month
"While we are postponing, life speeds by."
- Seneca