Movement variability and learning rate, running marathons, and measurement science
๐ Weekly paper summary
Category
Cross-sectional study (n=50)
Context
Movement is inherently variable due to the degeneracy afforded from having more degrees of freedom in joint space than in the workspace (often referred to as the degrees of freedom "problem"). How people exploit this degeneracy to accommodate task, environmental, and personal constraints is less well understood. Researchers have posited that individuals who demonstrate more movement solutions (i.e., more variability) can better exploit this degeneracy and might be more "flexible" with changing from one movement solution to another. Thus, these people may learn new tasks faster relative to those with less "flexibility." The central hypothesis for why people with more variability may learn more quickly is that those with more flexibility can better "sample" the solution space for the new task they are learning, and thus can "find" the appropriate solution faster relative to those who cannot sample the solution space with the same effectiveness. For more information on this general hypothesis (both in support and questioning the generality of the findings), please see Wu et al. (2014), He et al. (2016), Singh et al. (2016), and Haar et al. (2020).
There are two main gaps in the current literature that the authors addressed in this paper. The authors tackled a few gaps in their analysis. The central gap they addressed was that most previous studies have assessed how variability influences adaptation tasks, characterized by the participant making adjustments for constant errors (usually from perturbations or force fields) rather than the relatively permanent changes in underlying movement capacity of new skills. The latter typically reflects more real-world contexts of skill learning. Therefore, the purpose of this investigation (that I am choosing to summarise since the paper examined other data about the skill acquisition) is whether baseline movement variability was predictive of performance when learning a new movement solution. The authors hypothesized that baseline variability would be predictive of performance using the new solution.
Correctness
The authors did a good job selecting a task that would explicitly require a new solution. Participants performed a virtual shuffleboard task where they initially had to "throw" the puck virtually with both hands by grasping a bimanual "robot." Since the arms could move independently, participants could use multiple solutions to perform the task accurately (i.e., have the puck land at the center of the target). In the "novel" version of the task, participants were constrained to precisely aim the puck through a hole in a wall. The horizontal motion of the puck in this condition was determined by the difference in left versus right hand's velocity. With this task, a higher velocity with the right hand would move the puck to the right. Since the researchers placed the hole in the wall for the novel task on the right side of the screen, they were constrained to using specific solutions where the right hand was ~0.3m/s faster than the left hand. This constraint was a neat way of controlling participants to ensure that new solutions had to be adopted to perform the novel task.
The authors also had a reasonably large sample for this research area, which was nice to see. I also liked that the researchers decomposed variability into task-relevant and task-redundant (i.e., null) spaces, similar to uncontrolled-manifold approaches to decomposing movement variability into components that do or don't influence task accuracy. Finally, participants were aware of the "end goal" in the novel task in advance. In contrast, most studies intentionally make participants unaware of the transfer task in advance, and thus participants must experiment through trial-and-error to find a successful solution. Making the transfer task unclear to participants biases the need to have a large capacity for exploration, which may be problematic since people are aware of the "end-goal" when learning in most real-life learning scenarios. Therefore, this study did a good job controlling factors that might influence how these data translate into practical settings.
There were a few limitations of this analysis. First, participants performed all practice blocks in single learning sessions, which raises questions about how well these findings will generalize to other real-world contexts where learning occurs over weeks or months. Second, the authors operationalized "prediction" as to whether the variability presented at baseline correlates with the novel task's performance. The challenge with using a Pearson correlation for this purpose is that it assumes a linear relationship between baseline variability and performance of the novel task, which may not be a fair assumption. In my opinion, computing the mutual information between the two variables may be a better alternative, or at least an additional supplement to the Pearson correlation. A potential challenge with mutual information is that we need to know the probability distributions involved. Knowing the probability distributions of trial-to-trial movement variability is not necessarily straightforward either.
Contributions
Interestingly, the authors did not find that increased movement variability at baseline was predictive of performance on the novel task with the new solution. If anything, higher null space (task-invariant) variability was indicative of slower learning. Therefore, these findings raise questions about the generality of the assertion that increased movement variability facilitates quicker learning. One of the potential explanations for these findings presented by the authors is that the novel task in this experience was different enough that participants couldn't have "accidentally stumbled upon" the solution for the novel task but would have had to actively search for a new solution. These methods are different from most other studies where the new movement solution is typically much "closer" to the original solution. I think the authors sum up their main contribution at the end of the paper very effectively:
The observed variability in a given context is only a โsnapshotโ of the systemโs behavior and may not fully reflect the full potential of the system, which may explain why predictions using variability outside of the specific task context are likely to be less useful. Our results are consistent with the conclusion (He et al., 2016) that there is no single relation between variability and learning that generalizes to all contexts, and highlights the need for further work using tasks representative of real-world learning to fully understand the role of variability in motor learning (Haar et al., 2020)
๐ง Fun fact of the week
I've been running much more recently and am aiming to complete my first marathon sometime next year (hopefully around October). This week's fun fact, therefore, blew my mind.
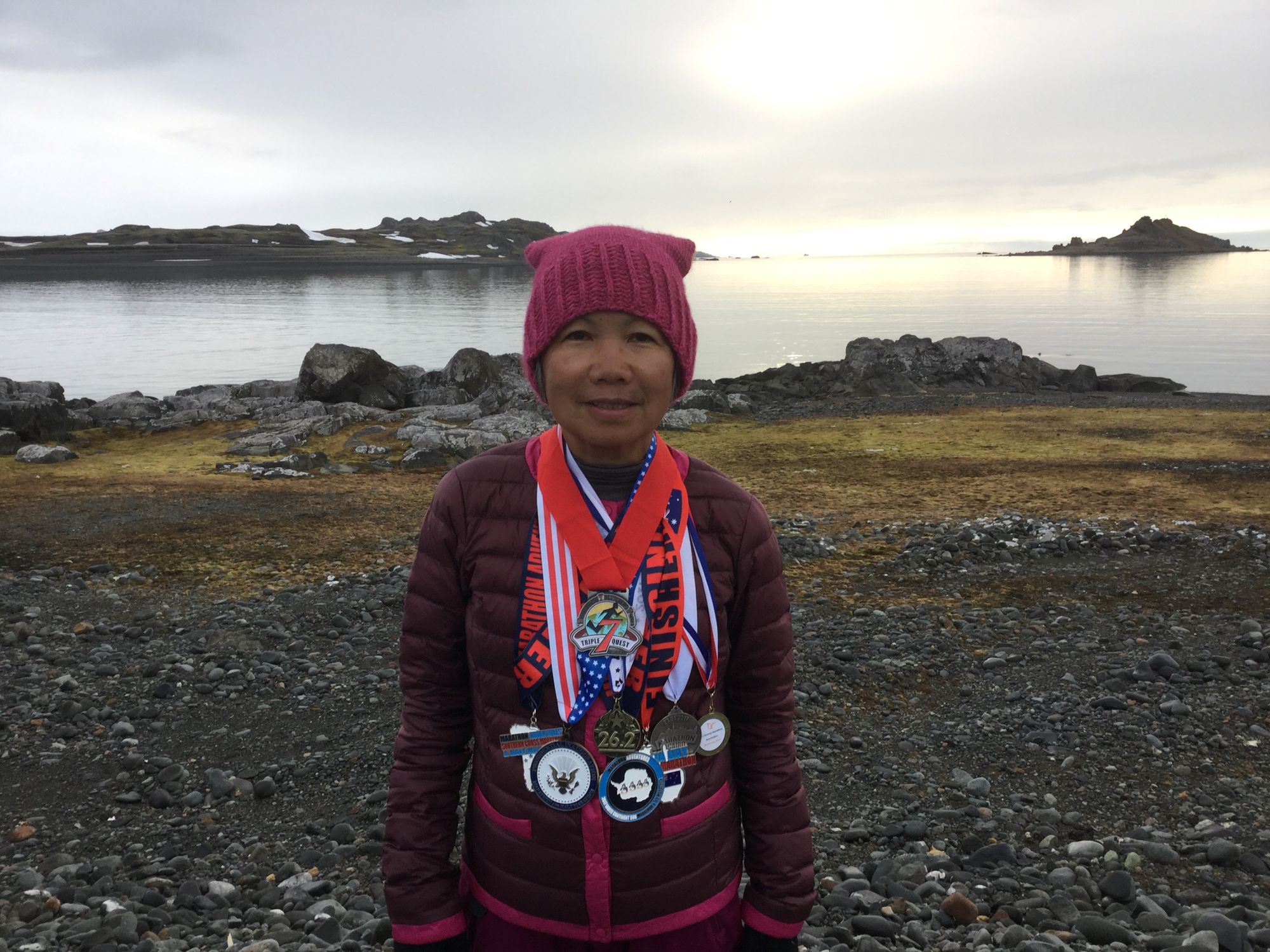
Chau Smith did not celebrate her 70th birthday like most people. Instead, she decided to run seven marathons for seven consecutive days on all seven continents. If you're interested in learning more about Chau and her story, check out this NBC article!
๐ Podcast recommendation
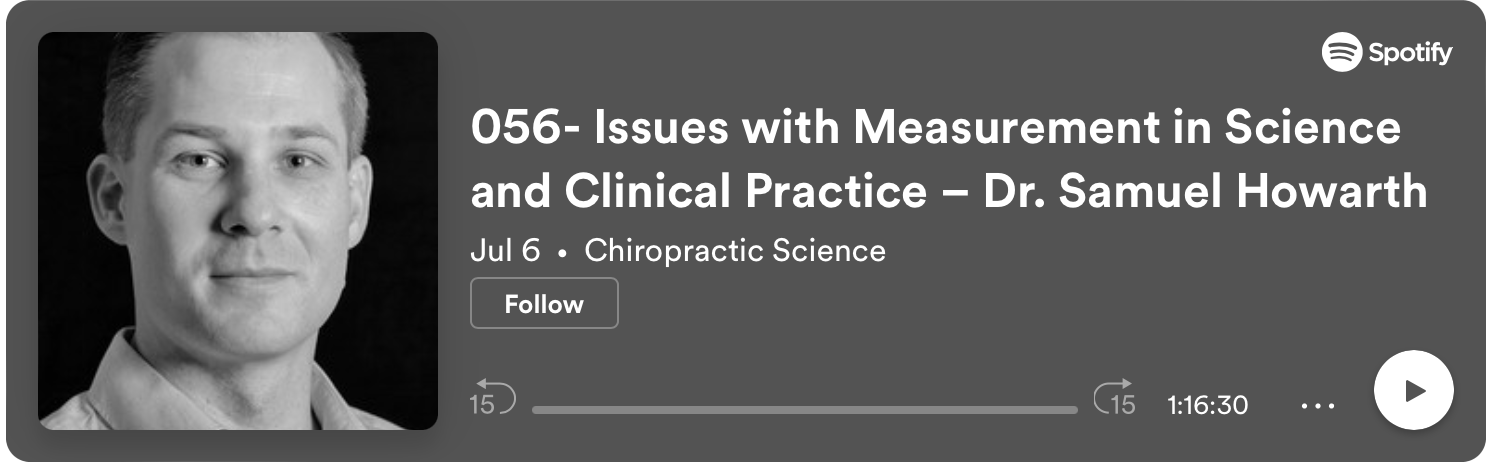
The title of this one says it all. Dr. Howarth does a great job outlining some current issues when making clinical measurements in science and practice.
๐ฃ Quote of the week
"We donโt rise to the level of our expectations, we fall to the level of our training."
- David Goggins