Machine learning and spine research, giant mushrooms, and inventing the future - October 12 Newsletter
📝 Weekly paper summary
Machine learning approaches applied in spinal pain research (Falla et al., 2021)
Category
Review article
Context
Low back pain (LBP) and neck pain (NP) are among the most significant contributors to spine pain and are the 4th and 9th leading causes of disability, respectively (as of 2019). Unfortunately, many of the proposed treatment plans to attenuate the prevalence of spine pain have been ineffective in the long term. One reason for this may be due to the variability between people. Thus, more research is required that accounts for clinical heterogeneity when developing and implementing assessments and therapeutic strategies.
The authors argue that the difficulty in translating the multifactorial nature of LBP and NP (i.e., accounting for the biological, psychological, and social factors influencing pain) is rooted in the challenge of managing and using high-dimensional multivariate data. The attraction of using machine learning techniques is to effectively integrate this multivariate data in ways that traditional linear models struggle. Therefore, these analytical approaches may offer unique insights that can help practitioners understand, harness, and translate the variability between people into practices that reduce the prevalence of LBP and NP. The purpose of this investigation was to review current pain mechanisms, evaluate current analytical strategies, reflect on the use of machine learning-based approaches in the literature, and provide a summary of the potential clinical implications from this review.
Correctness
I think the conclusions from this paper (outlined in the section below) support the general skepticism that many have over the potential impact of using machine learning in clinical settings. Numerous articles have outlined how machine learning hasn't really "lived up to the hype" yet. For example, IBM's Watson has famously overpromised and underdelivered on AI healthcare. Furthermore, Geoffery Hinton, regarded as one of the "fathers" of machine learning and winner of the 2018 Turing award, stated in 2016 that deep learning would outperform radiologists and render them obsolete within five years. As of 2020, only 30% of radiologists are currently using AI as part of their practice (Allen et al., 2021) (mainly due to poor performance; only 5.7% of respondents in this survey claimed that AI "always works" and 94.3% claimed inconsistent performance). Although one can rebuttal these findings and claim that we need more data to improve these algorithms, the field of deep learning is already stagnating due to the cost of improvement becoming environmentally and economically unsustainable. Therefore, although machine learning will undoubtedly yield improvements to society, I'm cautious about how much progress we might see in clinical practice after focusing so much on these analytical tools. For example, are any of the review's contributions novel? This paper, in some ways, reminds me of a tweet that I read recently:
https://t.co/4Ea9v9BOUr
— Ken Quarrie (@KenQuarrie) October 2, 2021
Hold on to your hats folks.
Researchers have discovered that *scoring is important in basketball* by using “machine learning” and “deep learning”. pic.twitter.com/8ChnX2ARFU
It's a bit sensational, to put it lightly (and there are other potential issues with what the authors call "deep learning" here). Still, it raises the question of what novel insights we are gleaning from these sophisticated approaches. I'm still somewhat cautious about how machine learning will contribute to scientific progress relative to conducting more and better experiments with larger sample sizes.
Contributions
- Early recovery phase pain trajectories indicate long-term clinical pain prediction (usually those with only a slight reduction in symptoms in the first six weeks experience more persistent pain). This finding isn't entirely surprising, but nevertheless comforting to know that our intuition of people's pain lasting longer if there is only a slight remission in the first six weeks is sound. For organizations looking to predict pain in workers (and thus days lost from work), this might be a powerful and simple way to project future costs.
- Static images of patients are much less capable of distinguishing those with and without pain than watching how someone moves.
- I loved Figure 5, which the authors adapted from Devecchi et al., 2020. Due to copyright reasons, I can't post it here, but when looking at the most important factors for predicting remission of NP, kinesiophobia was the second largest. This finding is important to highlight since regardless of what movement-related insights we glean from research, we don't want to make people afraid of moving either.
🧠 Fun fact of the week
About 350-420 million years ago, giant mushrooms covered the earth instead of trees. Imagine how that might have looked?
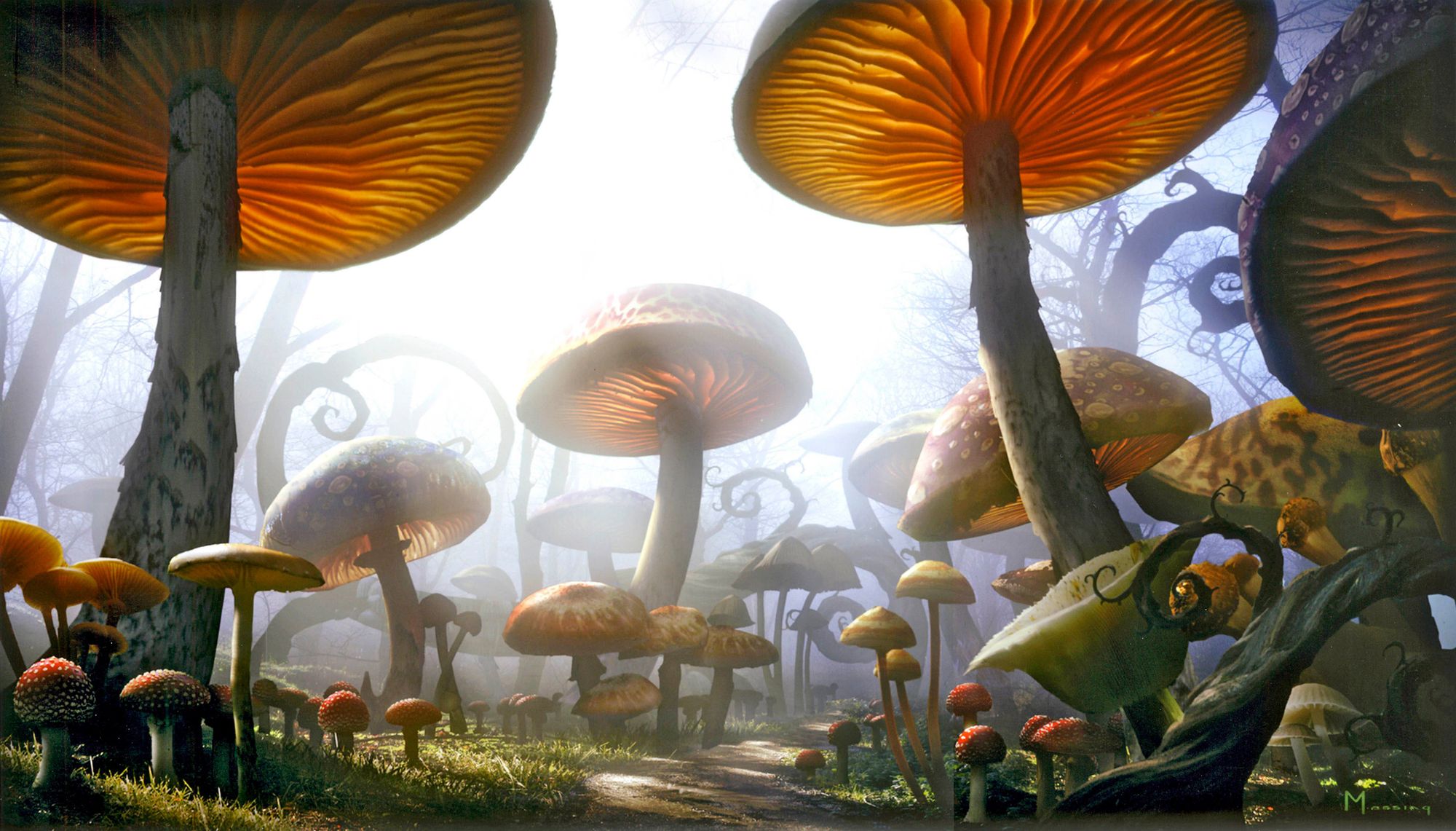
🎙 Podcast to check out
I found Sir James Dyson's story and lessons learned quite intriguing!
🗣 Quote of the week
"It's in keeping with Nature to show our friends affection and to celebrate their advancement, as if it were our very own. For if we don't do this, virtue, which is strengthened only by exercising our perceptions, will no longer endure in us"
- Seneca, Moral Letters, 109.15