Heavy back squats, More Markerless Motion Capture Solutions, and Thinking About Chess
🦾 Biomechanics, motor control, and (re)training paper recommendations
Motor Control: A Conceptual Framework for Rehabilitation

Much of rehabilitation and training hinges upon biomechanics and physiology with relatively little emphasis on motor control and learning. This paper by Mindy Levin provides suggestions for a unifying framework to understand motor control and motor learning, particularly in rehabilitation settings. However, I think many of the concepts also apply to strength and conditioning contexts!
A Generalised Smoothing Approach for Continuous, Planar, Inverse Kinematics Problems
Pohl et al. provide an interesting Bayesian Inverse Kinematics solution in their paper, with a nice GitHub repo accompanying it! For those unfamiliar, traditional Inverse Kinematics in biomechanics help to reduce measurement error in segment or joint positions by estimating the constrained pose (e.g., a model may have constrained joint angles and segment lengths) that is most similar to the empirically measured tracking markers from an experiment. For example, in markerless motion capture systems, it might be common for segment lengths to change from frame to frame since each frame of data is treated independently. An IK solver can estimate the "most likely" pose by minimizing the difference between the measured joint centers and a model that constrains the segment lengths (usually based on a least squares approach).
Setting up an Inverse Kinematics problem with a Bayesian approach allows for direct quantification of the uncertainty in estimated kinematics. The unique part of this paper is that it expands the Bayesian IK solver to account for the continuous time nature of human gait.
Effects of barbell load on kinematics, kinetics, and myoelectric activity in back squats
One particularly interesting thing to note from this paper is that they actually had a well-trained population with an average back squat 1RM of around 134kg! To my knowledge, this is also the largest published sample size that compares how people fail during their heavy back squats relative to how they complete their back squats.
🤖 Statistics and machine learning paper recommendations
MOVE Ai

The progress MOVE Ai has made recently in the markerless motion capture space has been absolutely insane. I think anyone interested in the general motion capture space should check out what they've been able to accomplish so far!
FMS Dataset with Two Kinect Azure Cameras
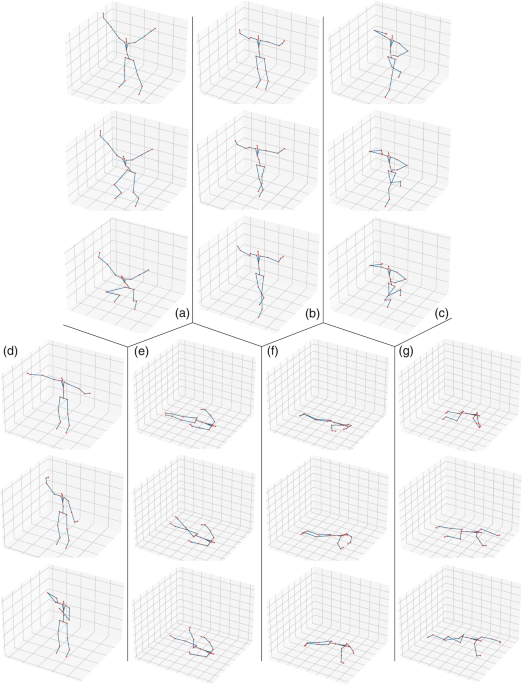
For those interested in any datasets for future data science-related projects, I'd recommend checking out this publically available dataset with FMS tasks! Their data can be downloaded using the following link.
DeepLabCut
DeepLabCut is another markerless motion capture solution worth checking out on GitHub! One really interesting thing is that it's a more general markerless motion-tracking model that can be used with various animals.
🎙 Podcast recommendation
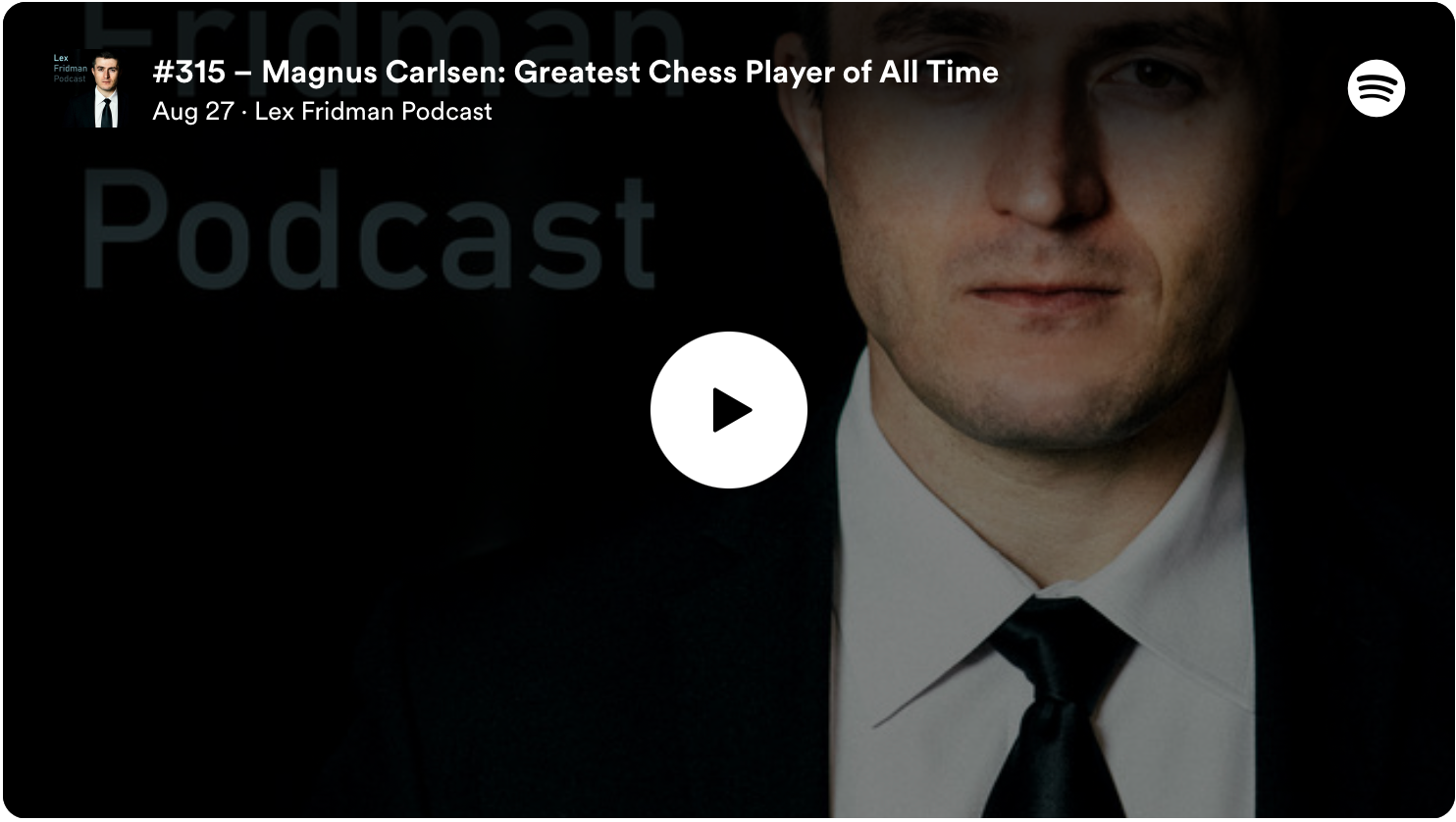
This one is relatively old, but I enjoyed listening to Magnus Carlsen on Lex's show.
đź—Ł Quote of the month
"In the military we always say we don’t rise to the level of our expectations, we fall to the level of our training"
- David Goggins
I've shared this quote before, but I think it's worth sharing again as it's particularly salient for me after completing my first-ever marathon!